In today's hyper-connected, digital world, the way we communicate has seen a significant transformation. From board meetings and doctor's visits to casual catch-ups, most of our conversations now occur online. As a result, the demand for recording and transcribing these digital interactions has skyrocketed. But with many speakers involved, accurately capturing who said what becomes a challenge. Enter speaker diarisation - a solution designed to address this very challenge.
Definition of Speaker Diarization

Speaker diarization is the art and science of distinguishing multiple voices in an audio stream and associating them with their respective speakers. In essence, it's about separating a mixture of voices into individual channels - one for each speaker. Imagine listening to a recorded Zoom meeting, and instead of a jumbled mix, each participant's contributions are clearly distinguished, just as if they were talking to you individually. That's speaker diarization at work.
Technological Complexities Behind Speaker Diarization
At first glance, speaker diarization might seem straightforward. But, delve a little deeper, and the complexities become evident. Achieving accurate diarization is no mean feat, requiring intricate technological models that can differentiate between nuanced differences in voices.
Several tech giants are at the forefront of this audio revolution. Companies like Rev, IBM, and Google are tirelessly working to enhance the accuracy of their diarization models, striving for perfection in an imperfect audio world.
How Speaker Diarization Systems Work
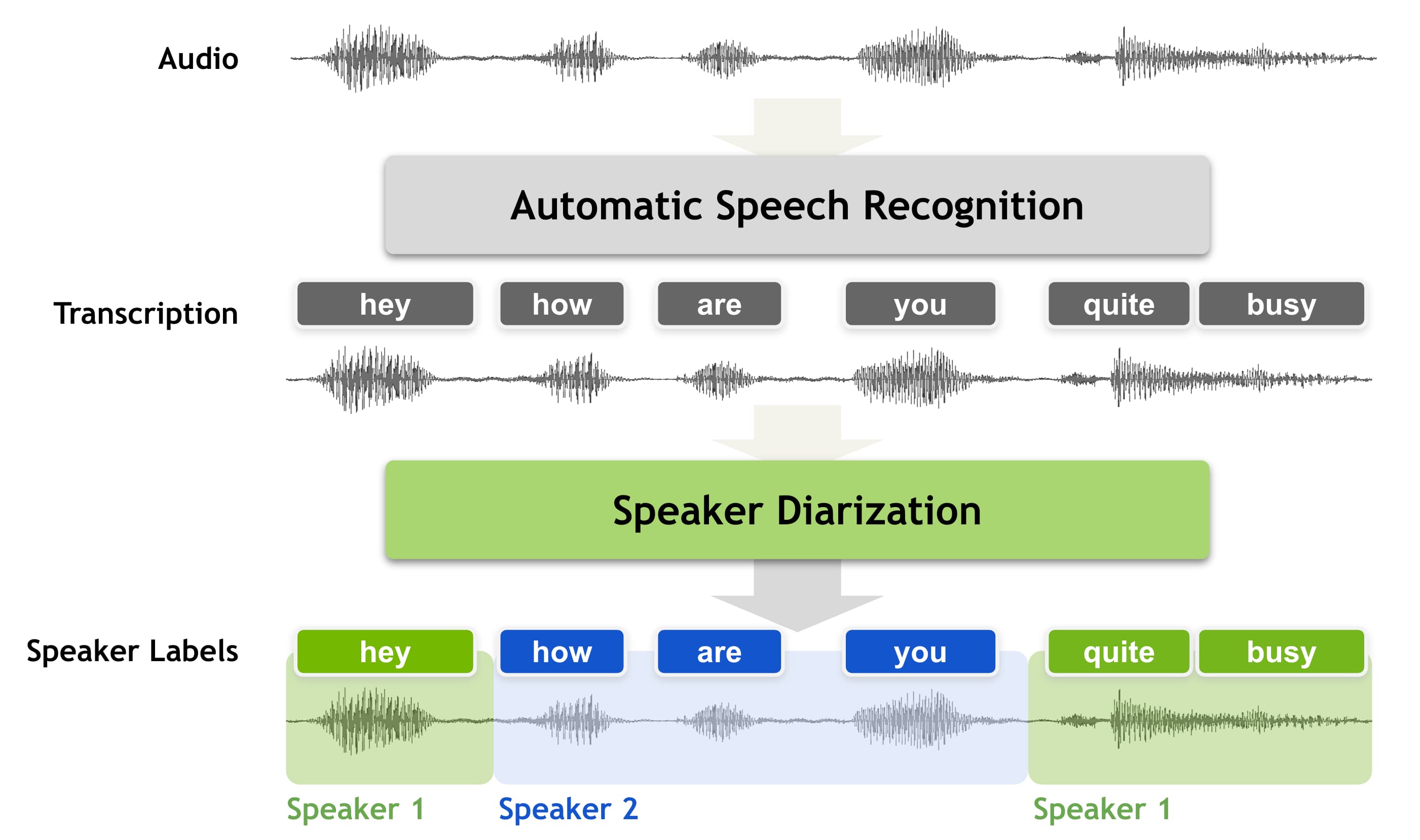
The road to accurate speaker diarization is paved with intricate steps. Let's break down the journey:
- Speech Detection: The initial step is to sieve out the speech from other noises. Using advanced algorithms, the system differentiates actual speech from background noises, ensuring only relevant audio is processed.
- Speech Segmentation: Once speech is detected, the next task is to divide it into small, manageable segments. These segments are created for each speaker, usually lasting around a second, forming the foundation for the subsequent steps.
- Embedding Extraction: With segments in place, the process then dives into creating a neural network for them. Each segment is embedded into this network, translating them into various data formats, from text and images to documents. It's like giving each segment its unique digital fingerprint.
- Clustering: Once embedded, the segments are grouped or clustered based on their unique characteristics. Similar segments, likely from the same speaker, are clustered together, ensuring each speaker's audio is grouped cohesively.
- Labeling Clusters: With clusters formed, they are then labeled. This labeling typically corresponds to the number of speakers identified in the audio stream, ensuring easy identification later on.
- Transcription: The final step sees these labeled clusters being transformed into text. The audio from each cluster is fed into a Speech-to-Text application, which meticulously transcribes the audio, resulting in a clear, speaker-distinguished transcript.
Common Use Cases for Speaker Diarization
Understanding the capabilities of speaker diarization can be a game-changer for multiple industries. Here's a breakdown of how various sectors are tapping into its potential:
- News and Broadcasting: For reporters and news agencies, speaker diarization is a boon. It aids in isolating individual voices during panel discussions, interviews, or debates, ensuring each speaker is accurately represented in the final broadcast or transcript.
- Marketing and Call Centers: Marketers and call center representatives are leveraging speaker diarization to gain insights from customer calls. Not only can they quickly identify the speaker, but they can also gauge sentiments based on voice modulations.
- Legal: Courtrooms and legal firms often deal with audio evidence or recorded testimonies. Speaker diarization helps differentiate between various voices, ensuring clarity in legal proceedings and documentations.
- Healthcare and Medical Services: In telemedicine or doctor-patient phone consultations, speaker diarization can prove invaluable. By distinguishing voices, medical practitioners can maintain accurate records of patient interactions.
- Software Development: As AI-driven applications like chatbots and home assistants become mainstream, developers need ways to decipher multi-user commands. Speaker diarization assists in distinguishing user voices, making device responses more accurate.
A Detailed Real-Life Example
Imagine this: A call center for a major retail brand handles thousands of calls daily. A customer calls to lodge a complaint about a recent purchase. The call involves the customer, a customer service representative, and eventually, a supervisor. Without speaker diarization, the transcription might read like a confusing jumble of voices. Who apologized? Who provided the solution? Who escalated the issue?
With speaker diarization, the transcript clearly demarcates each speaker. The brand can now effectively analyze the call, provide necessary training to its staff, or even identify recurring issues raised by customers. You can use speaker diarization feature of transcribetube in your transcriptions.
Why Software Developers Should Care

In the ever-evolving IT landscape, staying ahead of technological trends is imperative for developers. Speaker diarization is not just a trend; it's an essential tool. Here's why:
- Advanced AI Models: With tech giants like Google Brain and IBM pioneering real-time diarization capabilities, there's a wealth of knowledge and resources available for developers. Integrating these technologies can add immense value to applications and platforms.
- Rev's API Documentation: One such resource is the API documentation provided by Rev. It offers comprehensive insights into integrating diarization capabilities, ensuring developers have a roadmap to harness this technology effectively.
- Enhanced User Experience: In a world where voice-activated devices are gaining popularity, ensuring these devices can distinguish between multiple voices in a household or workspace can significantly enhance user experience.
Detailed Exploration of Speaker Diarization Subtasks
To fully grasp the depth and complexity of speaker diarization, we must delve deeper into its major subtasks. Each subtask contributes to the overarching goal of assigning individual labels to segments of audio streams.
- Detection: This initial step involves identifying and isolating instances of speech from non-speech segments. This means distinguishing spoken words from background noises, music, or silence. Robust detection ensures that only relevant audio sections proceed to the next stages.
- Segmentation: Once speech is detected, it is essential to chop the continuous speech stream into smaller, manageable chunks. These segments are created based on changes in the audio signal, ensuring that each segment contains speech from just one individual.
- Representation: In this phase, every segmented chunk is converted into a compact representation, often referred to as embeddings. These embeddings capture the unique characteristics of each speaker's voice, like tone, pitch, and speaking style, ensuring they can be distinctly recognized.
- Attribution: The final and crucial step is to group these embeddings (or segments) by similarity, thereby attributing them to individual speakers. Advanced clustering algorithms are employed here to ensure that segments from the same speaker are grouped together.
Significance of Speaker Diarization
Speaker diarization, though complex in its processes, offers very tangible and practical benefits:
- Enhanced Transcript Readability: Diarized transcriptions significantly enhance the reading experience. Instead of a jumble of words, readers get a structured dialogue with clearly identified speakers, making content digestion easier and more efficient.
- Real-World Applications: Consider sales meetings where multiple stakeholders discuss potential deals. With diarization, post-meeting reviews become straightforward, helping pinpoint individual contributions and concerns. Similarly, in online educational sessions with multiple participants, diarization aids educators in assessing individual student participation and responses.
FAQ About Speaker Diarization
1. What exactly is Speaker Diarization?
Speaker Diarization is the process of distinguishing and labeling different speakers in an audio file. Simply put, it tells us "who spoke when" in a given audio segment.
2. How does Speaker Diarization differ from transcription?
While transcription converts spoken language into written text, speaker diarization identifies and labels different speakers within that spoken content. Together, they can produce transcriptions where dialogue is attributed to specific speakers.
3. Why is Speaker Diarization important for businesses?
With the rise in virtual meetings, webinars, and conference calls, businesses need clarity on who said what. Speaker diarization provides structured dialogue, making post-meeting reviews, decision-making, and record-keeping more efficient.
4. Are there industries that benefit more from Speaker Diarization?
While many industries can benefit, areas like news broadcasting, call centers, legal proceedings, healthcare, and software development, especially in voice-activated assistants, find particular value in speaker diarization.
5. What challenges are currently faced in Speaker Diarization?
While the technology has advanced, challenges remain, such as handling overlapping speech, differentiating speakers with similar voices, and ensuring accuracy in noisy environments.
6. Can Speaker Diarization work in real-time?
Yes, tech giants like Google Brain and IBM have pioneered real-time diarization capabilities. This means that as words are spoken in a live setting, the system can identify and label speakers instantaneously.
7. How accurate is Speaker Diarization?
Accuracy varies based on the technology used and the quality of the audio file. Major tech companies have made significant strides, with some models achieving over 90% accuracy. However, results can vary based on the complexity and quality of the audio.
8. What's the future of Speaker Diarization?
As voice technology continues to evolve, we can expect speaker diarization to become even more accurate and integrated into a wider array of applications, from smart homes to more intelligent virtual assistants.
9. Does background noise affect Speaker Diarization?
While modern models are designed to be robust against background noise, extreme noise levels or multiple overlapping voices can pose challenges. It's always beneficial to have clear recordings for best results.
10. How can businesses integrate Speaker Diarization?
Many service providers offer APIs, like Rev's API, allowing businesses to incorporate speaker diarization into their existing systems seamlessly.
Related Blog Posts: